Speaker: Business Engineering Corporation
Solution Business Headquarters
SCM Solutions Division 2, SCM Solutions Department 3
Yohei Arumi
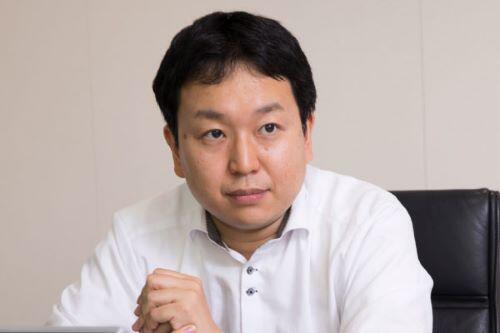
It is no exaggeration to say that reducing defective products to zero, or "zero defects," is an eternal theme for the manufacturing industry. The ideal situation is not to not ship defective products, but to "not make defective products in the first place."
To address this issue, the consulting team, led by Ari Kaihei of the SCM Solutions Division 2 of the Solutions Business Headquarters' SCM Solutions Division 2, is supporting quality improvement through analytical solutions that utilize machine learning, a core technology of third-generation AI (artificial intelligence).
Accelerating the PDCA cycle for quality improvement with a machine learning approach
One electronic parts manufacturer has continued to pursue zero defects as an important management theme, and as a result of daily quality improvements, they have achieved an average yield of 98% for their main products. However, eliminating the remaining 2% of defects before reaching the final goal is not easy. Even if they try every possible means, defects are caused by a combination of multiple factors, making it difficult to identify the root cause. In addition, these causes are sensitive to environmental changes and are extremely difficult to reproduce.
Amidst this, the team from the SCM Solutions Division 2 of the SCM Solutions 2nd Headquarters of the Solution Business Headquarters, with Ari Kaihei as project manager and consulting leader, has been in charge of consulting for this electronic parts manufacturer since March 2017, working together with the quality department of the production plant to resolve issues.
Specifically, the team led by Arumi is taking an approach that utilizes AI and machine learning to solve these problems. "Until now, customers have attempted to improve quality by applying statistical analysis methods to big data, such as data from prototypes and manufacturing history after mass production, that have been accumulated over many years. However, creating such mathematical models requires highly advanced and specialized academic knowledge, and it took a long time to derive results.
Therefore, the tool proposed by B-EN-G (*1) uses machine learning's Rule Induction method to interpret big data and discover the rules (commonalities) that produce good products and the rules that cause defective products. Because the analysis is fact-based, results can be obtained in a short time, and the PDCA cycle for quality improvement can be accelerated by simply 'following good rules and avoiding bad rules.'"
The most important requirement is to create a data management concept
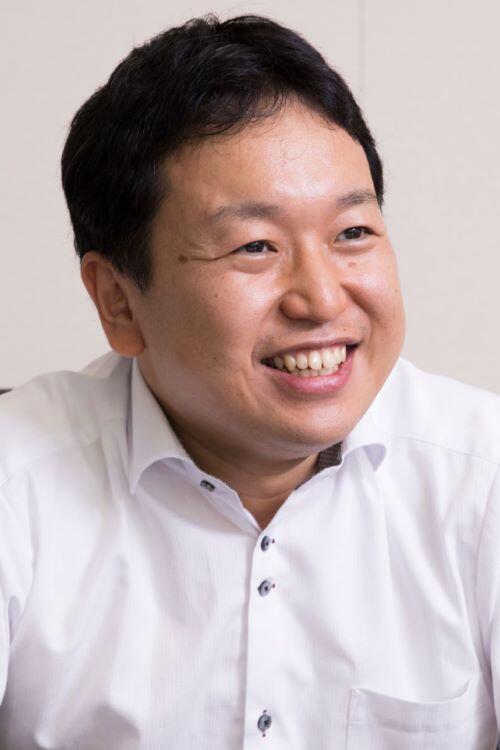
Machine learning approaches are by no means omnipotent. Even if you have a system for collecting big data and processing it quickly, the answers you seek will not appear automatically.
To begin with, even when we say data, the content is diverse. For example, the way text is entered varies greatly depending on the person in charge. Some numerical values are uniquely determined for each manufacturing process or lot, while others, like test data, include data for each sample and its statistical values (standard deviation, average value, etc.), and must be verified from multiple perspectives.
"How can we cleanse, consolidate, and integrate diverse data with completely different types, granularity, and specifications, and how can we ultimately visualize it in a control chart, correlation diagram, or other form? In other words, the selection of tools in the ETL (Extract Transform Load) area and the creation of a concept for data management itself are extremely important requirements."
Furthermore, the rules derived from analysis should never be treated as black boxes where results are all right. The root causes must be investigated from an academic perspective and backed up by theory.
"For this reason, we focused on the advantages of machine learning rule induction, which can create algorithms from big data and provide justification. By white-boxing data analysis, we can pinpoint the root causes that have a major impact on the occurrence of defects and then verify hypotheses."
Arumi's problem-solving strategy can be broadly summarized into the following four points.
1. Analysis can be performed even when the analytical method is not yet clear
Even if there are some exceptions, you can extract rules that look at the whole picture and get a rough idea of both good and bad trends. Based on those results, you can further analyze the results by going through the PDCA cycle.
2. You can utilize the knowledge of experts
It is possible to quantitatively evaluate using indicators that combine known rules of thumb from veterans at production sites who are familiar with manufacturing processes and parameters, combined with performance data. In addition, the system automatically detects conditions that should be added to improve the accuracy of the indicators.
3. Less susceptible to missing data
Since the conditional expressions for Good and Bad are created bottom-up and commonalities are discovered, it is less affected by the amount of data or missing data.
4.Various pattern analyses are possible
Because it is a methodology that is close to human thinking, it allows for free and diverse forms of analysis that are easy to put into practice in quality improvement work.
Utilizing quality improvement knowledge gained in the pharmaceutical industry
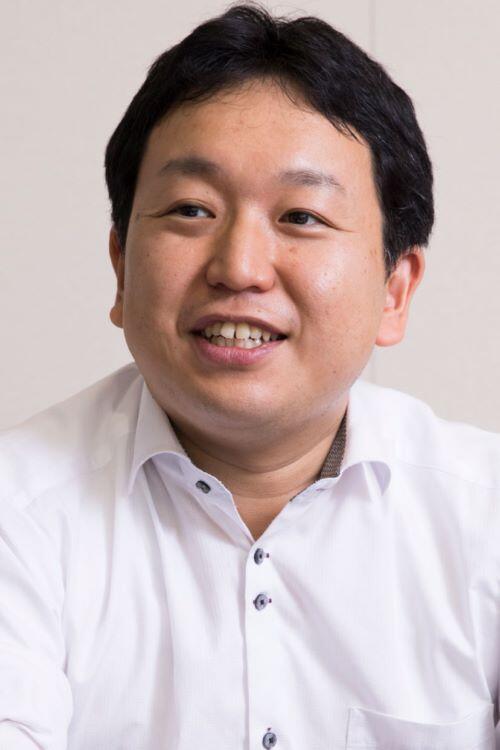
The reason why Arumi has honed his know-how in manufacturing quality data analysis as described above is largely due to his experience leading data integration and analysis to achieve plan compliance, stable operation, yield improvement, and danger detection, mainly in the production sector of the pharmaceutical industry.
We have supported consistent system integration, from ERP and MES (Manufacturing Execution System), to SCADA (Supervisory Control And Data Acquisition), which monitors the status of factory equipment such as sequencers and various inspection devices, and MHS (Material Handling System), which controls automatic transportation including warehouses.
Specifically, the company is using SAP Manufacturing Integration and Intelligence (SAP MII), which has a wide range of connectors, as a platform. By integrating manufacturing and quality data that was previously scattered across paper forms, personally managed Excel sheets, and multiple systems, the company has achieved online data collection, trend analysis of quality information based on indicators such as XBAR (average of measured values) and EWMA (moving average), and real-time monitoring via dashboards, which has made a major contribution to speeding up defective product investigations.
"The ability to grasp all data from the manufacturing site from the bottom up, starting from the equipment hierarchy, is something that has been passed down continuously since the days of the predecessor, Toyo Engineering's Industrial Systems Division, whose main business was plant engineering. Ultimately, we believe that this is a strength that B-EN-G cannot match. It is precisely because of this background that we are able to utilize the knowledge of quality improvement that we have cultivated in pharmaceutical production plants at an electronic parts manufacturer."
Of course, when the industry changes, the "language" exchanged among the people involved and the "common sense" that is tacitly known also differ greatly. How to adapt and respond to these changes is also an important point in consulting.
"Because I often have contact with quality control professionals (such as PhDs in engineering or pharmacy) at client sites, I am careful not to talk too much about systems. To do this, it goes without saying that I need to have a grasp of academic knowledge, such as statistics, which is the basis of the conversation, but I also try to get the latest information from articles and papers in the client's industry so that I can give more specific explanations."
This experience will then spread to the project team members and other departments in the Solution Business Division, further enhancing the overall strength of B-EN-G. This is also one of the strengths of B-EN-G.
*1: The underlying system used is Dassault Systèmes' DELMIA Operations Intelligence.
[The contents are current as of March 2018]