Speaker: Business Engineering Corporation
Solution Business Headquarters
SCM Solutions Division 2, SCM Solutions Department 3
Project manager
Keiji Tanaka
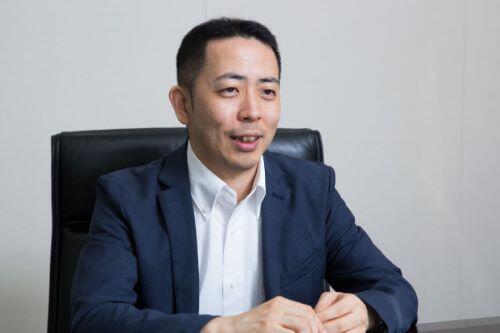
Many manufacturing companies have begun to take on the challenge of IoT, but the real challenges lie ahead after the system is put in place. It is easy to think that the right data will be collected if you simply connect various devices and sensors with the right protocols, but this is actually a big mistake.
Keiji Tanaka of the SCM Solutions Division 2 of the Solutions Business Headquarters, SCM Solutions Headquarters 2, who has been aware of this issue for the past 10 years and has been leading the vertical integration of "ERP-MES-workplace (equipment)" will explain how information sharing should be in the era of "Industrie 4.0."
Don't be under any illusions about IoT
Unexpected things happen one after another at the factory
Recently, the manufacturing industry has been actively working on IoT in anticipation of Industrie 4.0 (the fourth industrial revolution). From the perspective of manufacturing, this trend means that we have entered an era where it is no longer possible to gain the competitive edge needed to compete in the global market by simply repeating "kaizen" in areas that have traditionally been supported by MES.
In recent years, advances in IT in both hardware and software have made it relatively easy to build systems that collect data from various equipment distributed throughout a factory and from a variety of sensors placed at key points along a production line, and integrate the data on a single platform.
However, the real challenge would come "after that." A consulting team led by Tanaka Keiji of the SCM Solutions Division 2, SCM Solutions Division 2, Solution Business Headquarters, had already faced this challenge 10 years ago and had been trying to solve it.
The main setting for this project was an auto parts manufacturer A's catalyst manufacturing project that ran from 2007 to 2008. It seamlessly vertically integrated ERP, MES, and even the OT (Operational Technology) that manages and controls the site (equipment). By collecting a wide range of data, including production results, production conditions, and quality and inspection information, and linking and visualizing it in real time, the project achieved autonomous production with minimal manual work. This is the very theme of IoT that many manufacturers are currently trying to tackle.
Specifically, what issues have emerged from Company A's efforts? Many people tend to think that if they simply connect the equipment and sensor devices in a factory with the appropriate protocols, the correct data will be collected from each part at the scheduled timing (order, frequency). "Having such an illusion (expectation) is a mistake in the first place. After all, 'unforeseen' things happen one after another in a factory," says Tanaka.
"In particular, data generated by on-site equipment and sensors is often missing data, emitting outlying values that are far removed from reality, or arriving out of order. In other words, traditional single-task, sequential processing architectures are unable to handle this data appropriately."
Predict irregularities and error patterns
Building an automatic recovery (reprocessing) system
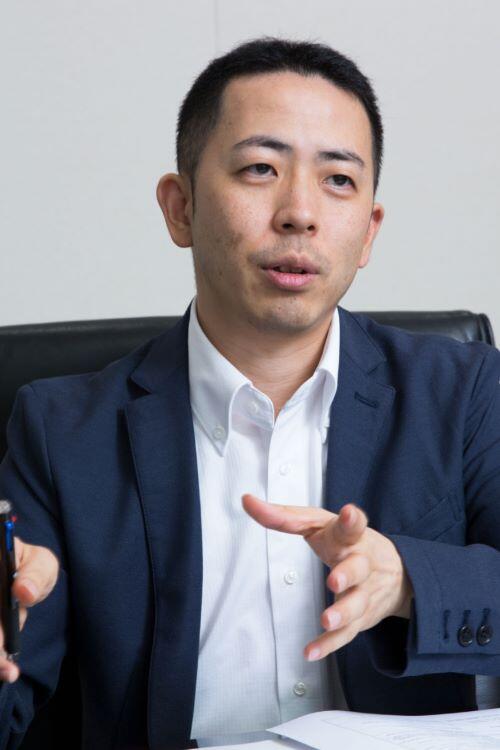
To solve the problems described above, it is necessary to identify in advance the wide variety of irregularities and error patterns that occur on manufacturing sites and then establish a multitasking, event-driven architecture that can respond flexibly to these patterns.
However, when it comes to uncovering patterns of irregularities and errors, the more devices and sensors that generate data there are, the more exponentially the number of combinations there will be, reaching a level far beyond the reach of human imagination.
To begin with, even when we say data, the content is diverse. For example, the way text is entered varies greatly depending on the person in charge. Some numerical values are uniquely determined for each manufacturing process or lot, while others, like test data, include data for each sample and its statistical values (standard deviation, average value, etc.), and must be verified from multiple perspectives.
For these reasons, many companies end up using MES-based data visualization. However, this only allows them to understand the progress of actual and forecasted output, the location of items, and superficial quality information about products.
However, Company A and the B-EN-G consulting team never gave up. They were determined to handle the big data generated from the vertically integrated ERP-MES-equipment environment.
In the midst of all this, it was Tanaka's experience that opened up a breakthrough.
"In fact, I was in charge of setting up new production equipment at a factory in my previous job. A total of 99 pieces of production equipment made up of more than a dozen multi-vendors were introduced all at once, and I was responsible for everything from formulating the specifications for each piece of equipment to deciding on the connection method and managing the progress of the project. Ultimately, I was responsible for putting together a system for collecting data from each piece of equipment. Through this experience, I had an ingrained sense that "irregularities and errors are normal for equipment to occur," and I also had prior knowledge of what kinds of things might actually happen. Thanks to this, I believe that even in Company A's project, I was able to predict the patterns of irregularities and errors that could occur on-site relatively smoothly."
Based on Tanaka's knowledge, the B-EN-G consulting team solved the problem by building a system that automatically recovers (reprocesses) data when irregular data or errors occur. Ultimately, autonomous production was achieved. Company A's vertically integrated ERP-MES-equipment environment continues to evolve even today, with the repeated introduction and replacement of new equipment.
"Now, in the unlikely event that a problem (recall) occurs after shipment, we are able to quickly identify the affected product and the cause from the data we have collected and accumulated. We are also focusing on collecting a wider range of data surrounding the manufacturing process. For example, we are able to grasp in real time the manufacturing conditions of each piece of equipment (parameter settings, temperature, pressure, air volume during operation, etc.) as well as changes in environmental information (temperature and humidity) at the manufacturing site. We are also able to link this data to BI analysis tools, which leads to new discoveries."
Furthermore, Company A is planning to expand its production line in the spring of 2018, and by applying the standard templates that it has developed based on its track record to date, it will be able to flexibly respond to the introduction of new equipment and the expansion of data.
Because the data is collected on-site
Information deployment from design to planning, procurement, and manufacturing
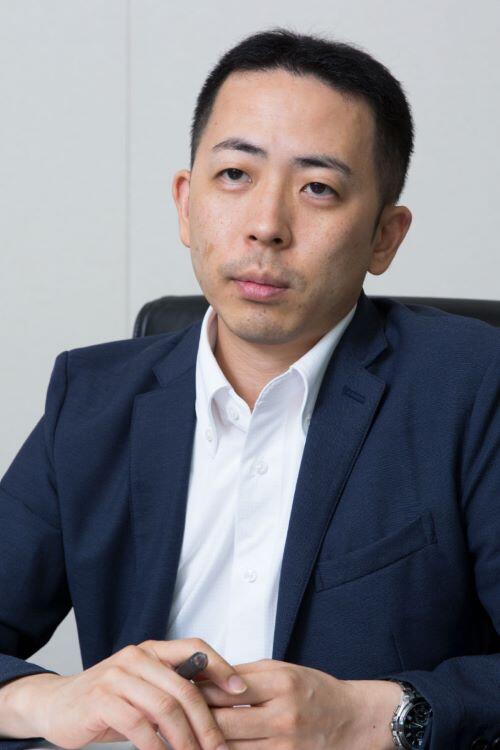
Of course, there are still many challenges facing the Japanese manufacturing industry, and this is not limited to Company A. Just as they have been looking ahead to the current IoT challenges for the past 10 years, they are now looking ahead to the challenges that lie ahead in the next 10 years.
One of the themes is the distribution of information from design to planning, procurement and manufacturing.
"In particular, in response to changing customer needs in global markets, shorter lead times, and demands for quality improvement, it is important to share the intentions and goals of the design side with the field, and then provide feedback from the field to the design side. The key is to increase the speed and smoothness of this two-way information transfer. This is the core element advocated by Industrie 4.0, and it will be an extremely effective way to establish manufacturing methods such as 'mass customization' and 'personalization' ahead of our competitors."
In fact, even today, it is not uncommon for design and the workplace to be separated in the manufacturing industry, which leads to inefficiencies in production. "For example, a manufacturing line designer always has in mind the 'ideal state' of how the equipment and workers that make up the line should operate. Only when this information is shared and understood by the workplace can the 'awareness' emerge about what data should be collected and what visualization and monitoring should be performed in order to get closer to that ideal state. Furthermore, data collected on-site based on this perspective can also be used in manufacturing line design. Naturally, this data is also used in product development and design."
In response to the challenges that many Japanese companies are expected to face in the process of moving towards IoT, Tanaka and the B-EN-G consulting team are proactively preparing a methodology for design-on-site collaboration, as well as a basic KPI set to support the initiative, a standard template for data sharing, and analytical algorithms using AI. They intend to establish a system that can "always respond" to the requests of all manufacturing industries, regardless of whether they are in the discrete or process industry.
[The contents are current as of January 2018]